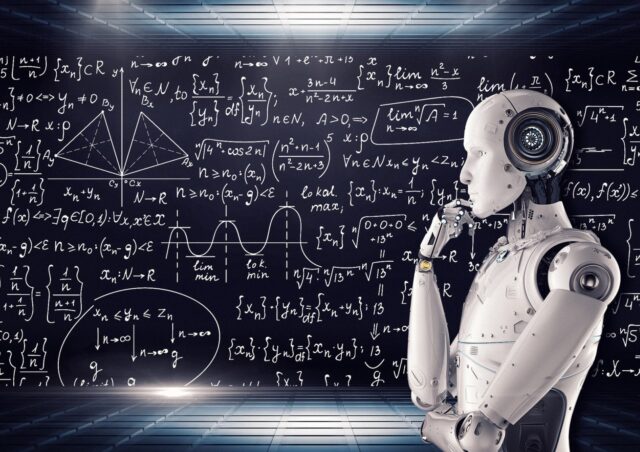
There has been lots of buzz around the topic of machine learning (ML) in recent years. Although this concept isn’t really new, today, it is literally everywhere.
As technological progress stepped further, ML became an integral element in business, research, and numerous other industries. Some examples of its use in the real-life are:
- Speech recognition
- Image recognition
- Medical diagnosis
- Statistical arbitrage, etc.
Though people are talking about machine learning a lot, not everyone understands what it is and how it works quite right. And it’s quite natural. The concept of ML is rather complex. Thus, if you were assigned to write an essay on this topic, we recommend turning to EssayHub for help. There you can get a cheap essay and rid yourself of unnecessary stress and work.
But, if you are determined to puzzle out this matter, in this article we are going to help you understand how machine learning differs from human learning (HL). Let’s dive in!
The Difference Between Machine Learning and Human Learning
The core purpose of learning is generating knowledge. Both ML and HL do just that – they generate new knowledge. But, does this mean that there are no differences between them? Not at all!
Clearly, humans are very different from machines. But what exactly makes ML and HL so different?
Basic Process in ML and HL
First of all, it is worth noting that there is no one-fits-all definition of human learning. That is, this process and steps included in it vary from person to person. What’s more, with time, these processes transform into habitual patterns that are really hard to change once they are set into the mind.
For example, if you are used to checking out reviews about the products or services before you use them, you will most likely do the same regardless of the type of product. That is, even if you have never used an essay writing service before, when you decide to use one, you will most likely look for essay writing services reviews before choosing a service that suits your needs. That’s just how the process is set in your mind.
In this sense, ML is much different. A machine also has a well-defined learning process that is programmed into it. But, this process is easy to change. All you need is to input a different algorithm.
Analogical Reasoning and Critical Thinking
One of the main hallmarks of HL is that we rely heavily on analogical reasoning and critical thinking. When facing a specific problem, people tend to employ analogies across different domains to find a reasonable solution. This is something machines still can’t do. They are often programmed to solve only specific types of problems and rely more on associations instead of making analogies.
Ability and Speed of Acquiring, Processing, and Retaining Information
Here is one more difference – people start learning by memorizing basic concepts and words, which typically happens in early childhood. Then, year by year, we practice transforming the information we already have stored in memory into knowledge and practice to apply it to solve various problems and develop skills.
This also implies that not all people have the same level of intelligence. Our intelligence is defined by a number of factors such as memory, skills, knowledge, and also personal traits. All these factors influence our ability to acquire and retain new information (as well as the speed of learning) and retain knowledge.
Machines, on the contrary, are exposed to huge amounts of data from the world wide web by default. The retention possibilities, of course, are larger than in humans. Whereas all concomitant abilities, including the speed of learning, are determined by the volume of available data and selected algorithms.
Sources of Knowledge
Another difference resides in the sources from which knowledge is acquired. In particular, people can acquire knowledge through personal experiences or experiences shared by others. Machines also collect knowledge through experience. However, in this case, the experience is shared in the form of past data.
Volumes of Data
Needless to say that a person’s ability in terms of volumes of data that is being processed and retained is much weaker than it is in machines. Thus, even though machines are mimicking the processes inherent in the human brain, their power is much larger.
Assessment of the Accuracy of Acquired Information
In humans, we can only estimate the accuracy and level of knowledge through various examinations and it is worth noting that it can’t be considered as an indication of intelligence. In the case of machines, the accuracy of learning can be estimated with the help of well-defined processes and algorithms.
General Intelligence
Getting back to intelligence, one more thing that makes us different is that our intelligence is manifested through our skills (i.e. the ability to apply knowledge to solve different problems). Although our knowledge may fade or we may not possess knowledge in a particular field, intelligence sustains. That is, people may apply their intelligence to solve problems in various new domains even if they don’t have prior knowledge related to them.
Things are different with machines. In order to solve problems in new domains, their intelligence needs to be updated and retrained. At this point, this is one of the most fundamental distinctive features between ML and HL.
The Bottom Line
As you now know, despite certain similarities in processes, functions, and purpose, ML and HL are very different.
Though computers can process larger pools of data and the accuracy of their knowledge is easier to measure, they can’t solve problems in new domains without retraining. This suggests that computers cannot replace the human brain completely. At least, not at this point.
So, how do we apply these knowledge resources? With so many differences, it is fair to say that the best way to reach optimal results is to find a balance between machine and human learning. Though each of them may have certain flaws, together, these knowledge resources can give us almost unlimited opportunities in pretty much any industry and subject matter.